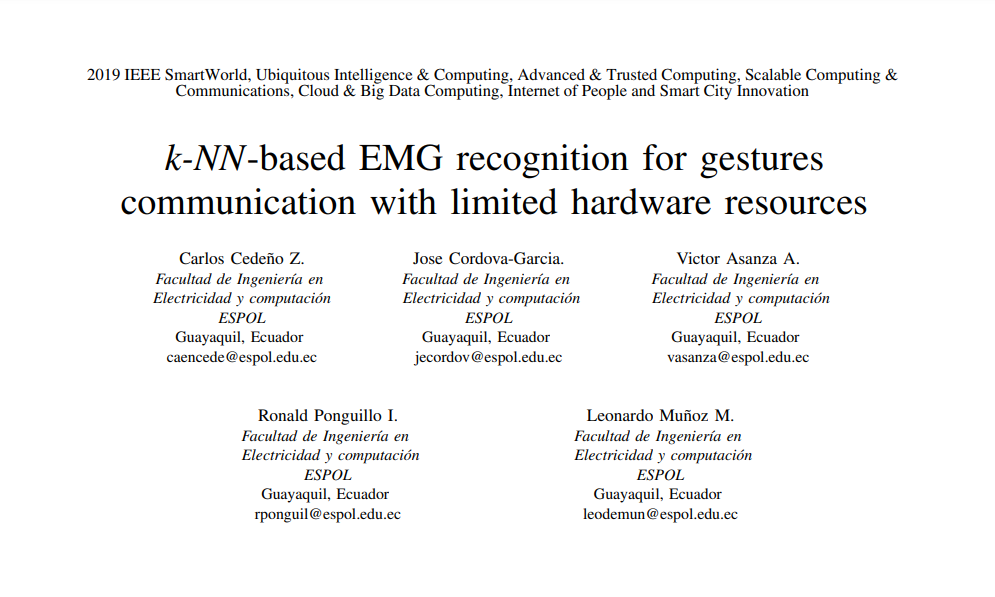
k-NN-Based EMG Recognition for Gestures Communication with Limited Hardware Resources
Abstract: Analysis of Electromyographic signals (EMG) allows obtaining useful information to develop gesture recognition applications. In this paper we propose an FPGA-based wearable EMG gesture recognition system to support the communication of customized phrases for people with speech impairments. Machine learning algorithms can accurately identify gestures, usually relying in a large number of features or large training datasets. In this work, we focus on using a small number of features in the gesture classification process in order to reduce the need for computational power. Namely, we propose using only one feature, the Root Mean Square (RMS) signal value, and the KNN supervised classification algorithm. The system is evaluated in an DE10-Standard FPGA to demonstrate portability to wearable devices with limited hardware resources. Tests show that subjects only need three seconds per gesture to train the system, which avoids processing large amounts of data and improves user experience during the equipment setup. Furthermore, the accuracy of the system reaches 95% using only 2 seconds of data effectively maintaining both human and hardware resources low.
View Paper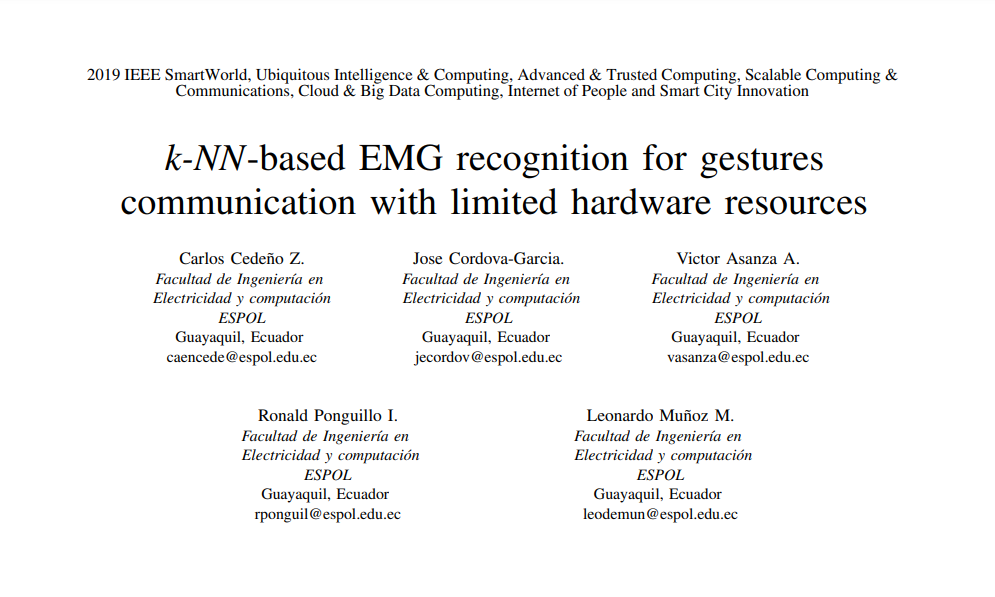
k-NN-Based EMG Recognition for Gestures Communication with Limited Hardware Resources
Abstract: Analysis of Electromyographic signals (EMG) allows obtaining useful information to develop gesture recognition applications. In this paper we propose an FPGA-based wearable EMG gesture recognition system to support the communication of customized phrases for people with speech impairments. Machine learning algorithms can accurately identify gestures, usually relying in a large number of features or large training datasets. In this work, we focus on using a small number of features in the gesture classification process in order to reduce the need for computational power. Namely, we propose using only one feature, the Root Mean Square (RMS) signal value, and the KNN supervised classification algorithm. The system is evaluated in an DE10-Standard FPGA to demonstrate portability to wearable devices with limited hardware resources. Tests show that subjects only need three seconds per gesture to train the system, which avoids processing large amounts of data and improves user experience during the equipment setup. Furthermore, the accuracy of the system reaches 95% using only 2 seconds of data effectively maintaining both human and hardware resources low.
View Paper